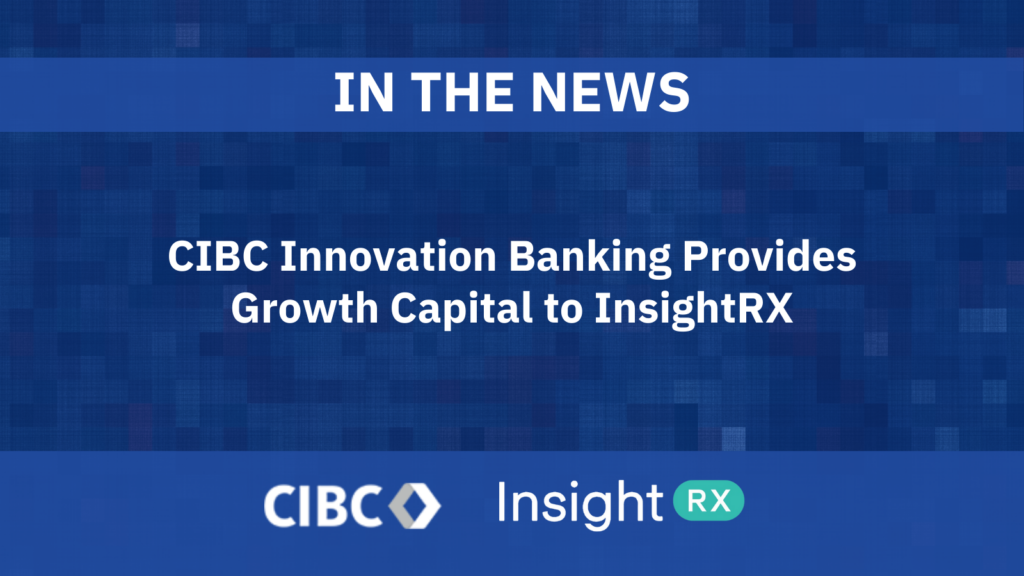
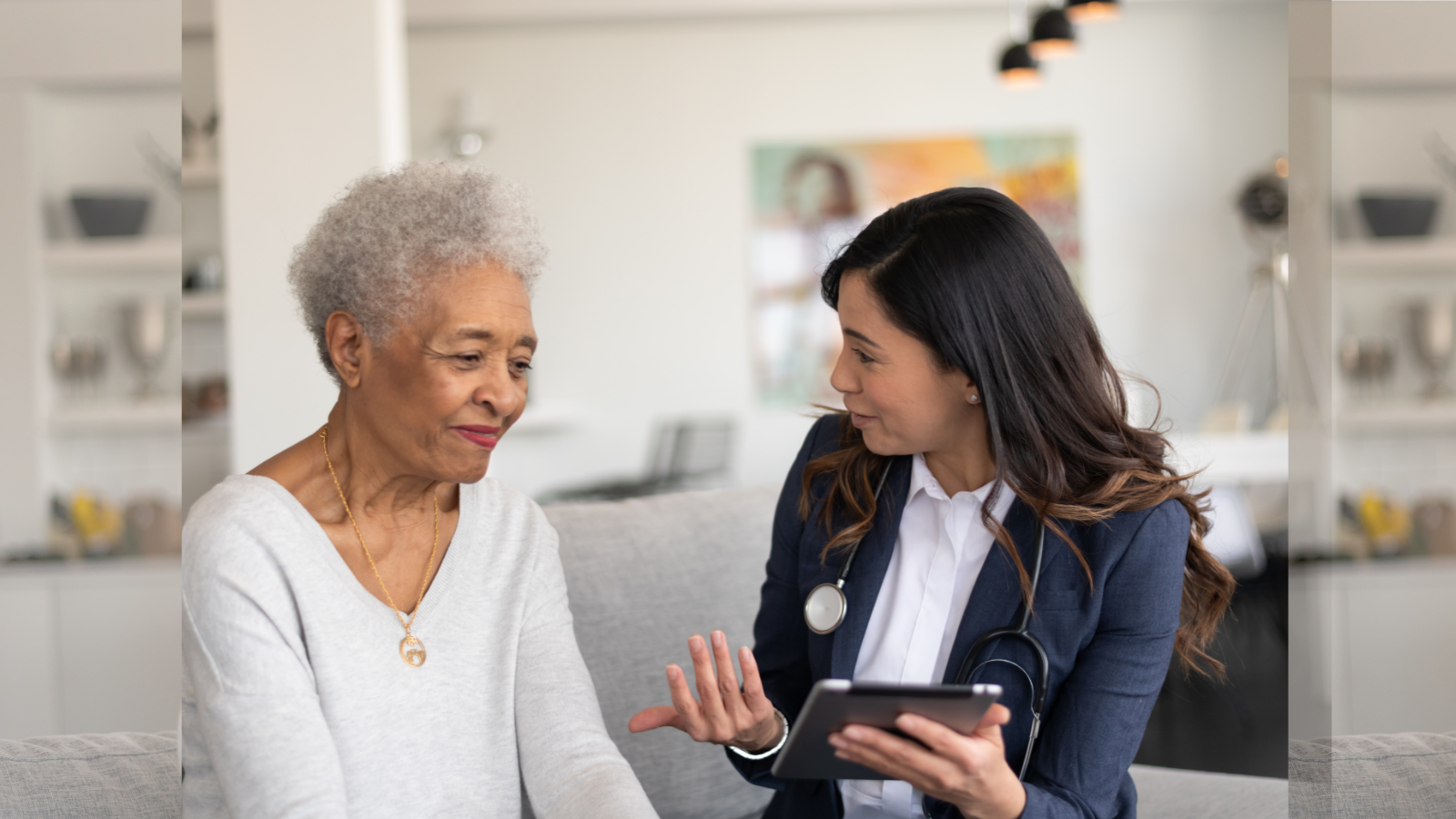
How Clinical Decision Support for Precision Dosing Can Enhance Healthcare Equity and Inclusion
Clinical decision support software (CDSS) sits at a critical juncture in precision medicine. Research efforts over recent decades have accumulated massive amounts of knowledge about how patients respond to medications, however translating these gleanings to the patient bedside has been challenging. CDSS consolidates and presents this knowledge in ways that clinicians can use at the point-of-care to inform how they treat their patients. Because of the crucial role CDSS plays in patient care, it presents a valuable opportunity for enhancing inclusion and equity in healthcare.
Clinical Pharmacology & Therapeutics (CPT), a peer-reviewed journal of the American Society for Clinical Pharmacology and Therapeutics, published a special issue centered around Diversity, Equity and Inclusion (DEI). We were proud to be invited by this prestigious journal to contribute a review paper describing how CDSS for precision dosing can enhance DEI. Part of our mission at InsightRX is to use our technology to improve equity and inclusion, so it’s gratifying that CPT editors are thinking about our company in this particular space.
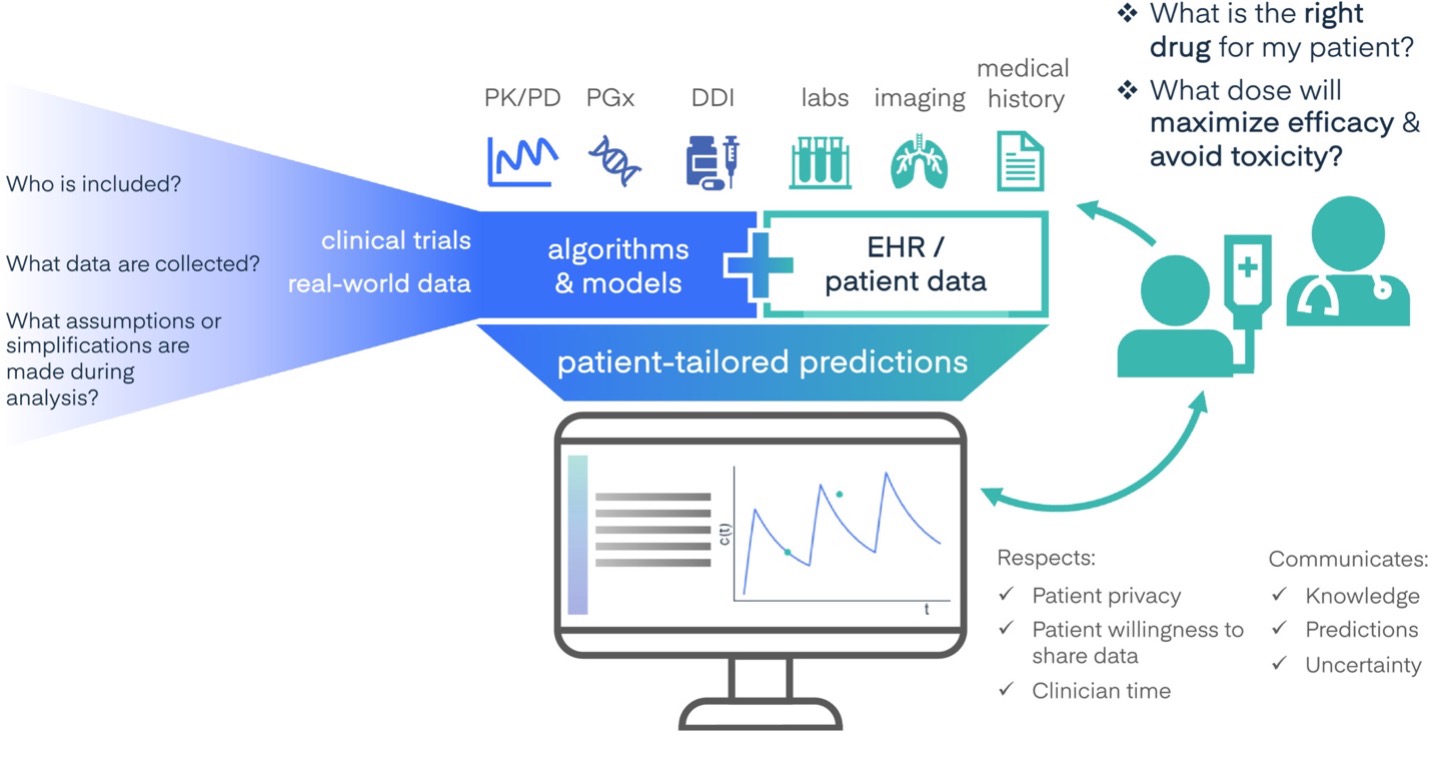
A major theme we focus on in the paper is that while CDSS tools can translate knowledge derived from clinical trials and real-world data (RWD) into actionable clinical insights, not all patient populations are proportionally represented in the data sources that inform CDSS tools. This limits the applicability of these tools for underrepresented populations. As we write in the paper: The precision medicine revolution has not advanced our understanding of medicine in all populations equally. Genomic and pharmacogenomic studies have overwhelmingly been conducted in patients of European ancestry. Some patient populations, such as pregnant or paraplegic patients, are regularly excluded from clinical trials and postmarket studies due to difficulties they present in data collection or modeling. Furthermore, models and CDSS often rely on discretized characteristics of patients—binarizing sex or gender, or reducing patient ancestry to one of a handful of categories. These simplifications complicate clinical decision making for patients that do not neatly fit within these categories—such as transgender, intersex, or biracial patients. The lack of underrepresented populations in research studies is felt at the point of care. One example we discuss in our paper is the use of race in heuristics intended to estimate renal function. These equations found that race alters the relationship between biomarkers and kidney function, but it is likely that race acted as a proxy for existing health disparities related to socioeconomics rather than biological phenomena. The use of such biased equations can underestimate chronic kidney disease risk, preventing Black patients from receiving certain treatments. In such cases, technology inadvertently perpetuates racial disparities in healthcare.
We also cite examples of how RWD and CDSS are being used to continuously improve drug dosing recommendations following the approval of a treatment. RWD includes patients’ EHRs, insurance claims, disease and product registries and genomic data, and better reflects the diversity of the “real world” compared to populations studied in clinical trials. By leveraging CDSS tools to collect RWD during the course of routine clinical care, researchers can create an ongoing feedback loop in which algorithms are continually learning from the RWD. This RWD data can offset the lack of diversity found in clinical trial data due to nonparticipation by underrepresented groups.
However, the example of estimation of renal function is an important cautionary tale; biases present in data and decisions made during the analysis process can create biased models. In our paper, we provide recommendations for model developers, study designers and software interface designers for how to reduce bias in data collection and algorithm development. Among our suggestions is that developers of CDSS systems “create interfaces that reflect patient diversity and gracefully handle missing data” while offering speed, integration into clinicians’ workflows, and clear recommendations and guidance.
I want to thank the editors and reviewers at CPT as well as my InsightRX colleagues and coauthors – CEO Sirj Goswami, CSO Ron Keizer, and Senior Data Science Engineer Kara Woo – for enabling this public discussion of how CDSS for precision dosing can enhance equity and inclusion in healthcare.