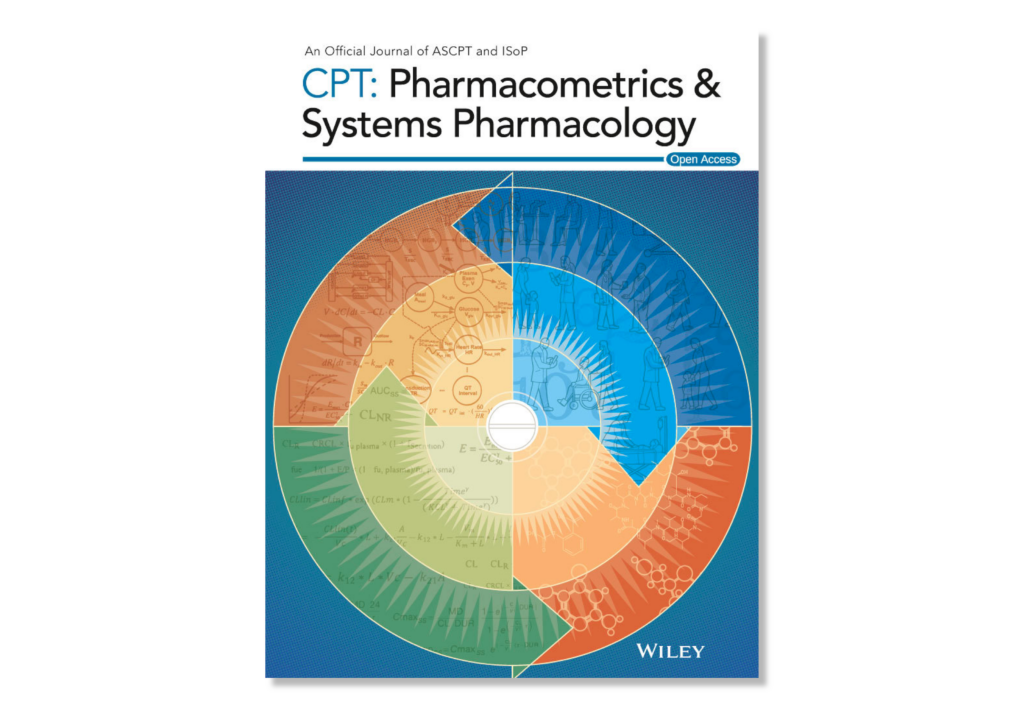
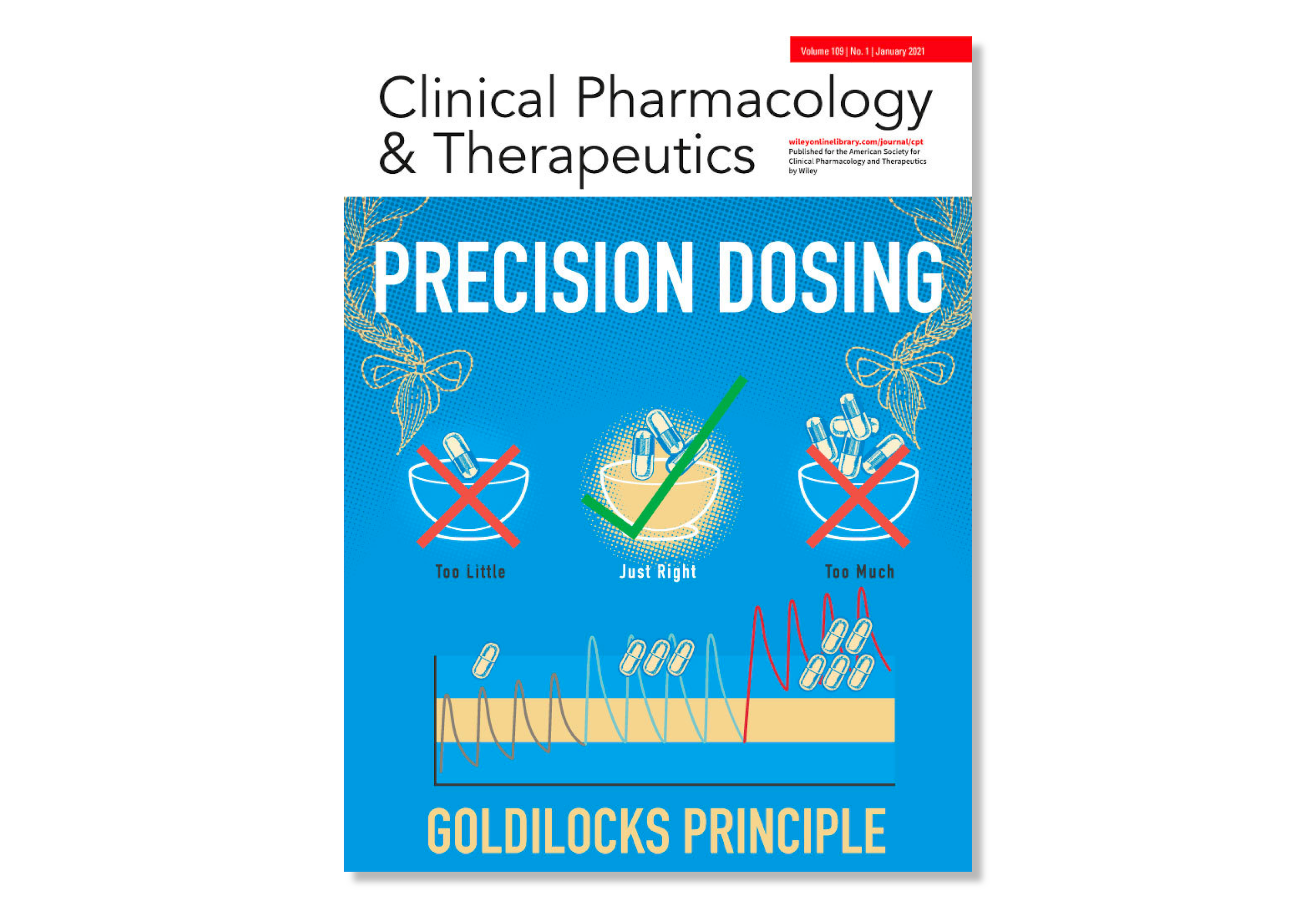
Continuous Learning in Model-Informed Precision Dosing: A Case Study in Pediatric Dosing of Vancomycin
Abstract
Model-informed precision dosing (MIPD) leverages pharmacokinetic (PK) models to tailor dosing to an individual patient’s needs, improving attainment of therapeutic drug exposure targets and thus potentially improving drug efficacy or reducing adverse events. However, selection of an appropriate model for supporting clinical decision making is not trivial. Error or bias in dose selection may arise if the selected model was developed in a population not fully representative of the intended MIPD population. One previously proposed approach is continuous learning, in which an initial model is used in MIPD and then updated as additional data becomes available. In this case study of pediatric vancomycin MIPD, the potential benefits of the continuous learning approach are investigated. Five previously published models were evaluated and found to perform adequately in a data set of 273 pediatric patients in the intensive care unit. Additionally, two predefined simple PK models were fitted on separate populations of 50–350 patients in an approach mimicking clinical implementation of automated continuous learning. With these continuous learning models, prediction error using population PK parameters could be reduced by 2–13% compared with previously published models. Sample sizes of at least 200 patients were found suitable for capturing the interindividual variability in vancomycin at this institution, with limited benefits of larger data sets. Although comprised mostly of trough samples, these sparsely sampled routine clinical data allowed for reasonable estimation of simulated area under the curve (AUC). Together, these findings lay the foundations for a continuous learning MIPD approach.
Hughes, J.H., Tong, D.M., Scarpace Lucas, S., Faldasz, J.D., Goswami, S. and Keizer, R.J. (2021), Continuous Learning in Model‐Informed Precision Dosing: A Case Study in Pediatric Dosing of Vancomycin. Clinical Pharmacology & Therapeutics. Accepted Author Manuscript. doi:10.1002/cpt.2088